Does Data Science Want Statistics?
- robingilll295
- Sep 3, 2021
- 6 min read
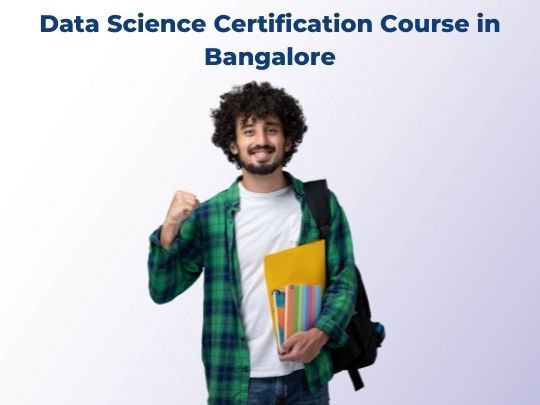
Then, they use their information of social sciences and specific industries or sectors to interpret the means of these patterns and how they apply to real-world situations. In practical conditions, statistical inference can involve both estimating a population parameter or making decisions in regard to the worth of the parameter. The latter involves asking a “hypothetical” query in regards to the data population and finding the reply by testing a small pattern data. An information scientist ought to know the means to effectively use statistics to gain insights from information. Here are five useful and practical statistical concepts that each information scientist should know. This gives consideration to small information explains why it is necessary to quantify uncertainty in statistics.
Plus our Career Services team will work with you to ensure you don't solely be taught the talents you need, but you land the job once you do. You’re going to bump up alongside the perimeters of data principle fairly typically whereas learning data science. For many people with traumatic experiences of mathematics from high school or school, the thought that they’ll re-learn calculus is a real obstacle to becoming a data scientist. In this publish, we understood why and how statistics are important to know and work with information science. There are other distributions as properly, and it depends on the kind of data we've and the insights we need from that data, however, Gaussian is taken into account as one of many fundamental distributions. The concept right here is to get the value of the posterior likelihood, given other data. The posterior likelihood for quite a lot of completely different hypotheses must be found out, and the probability that has the very best value is selected.
When we discuss developing insights out of knowledge it's mainly digging out the chances. Those prospects in Data Science are often recognized as Statistical Analysis. Introduction to Statistical Machine Learning is a beautiful textbook that you can use as a reference. The examples are in R, and the book covers a wider range of topics, making this a valuable tool as you progress into more work in machine studying. The following models have been chosen because they illustrate a number of of the key ideas from earlier. For instance, data scientists usually have to resolve which results are believable and which are bullshit likely because of randomness.
In this method, you mainly construct a statistical mannequin and broaden it for the whole population in the class. But, you want to never, ever fully skip studying statistics and likelihood theory. It is a technique that helps in finding the values of parameters for a selected mannequin.
While such data typically happens in statistics, it's the exception somewhat than the norm. Historically, the give attention to statistics has been far more about what can be realized from very small quantities of information. To conclude the demo, we’ll be plotting a graph for each continent, such that the graph exhibits how the life expectancy for every continent varies with the respective GDP per capita for that continent. Notice the imply in group Ireland and in South Africa, you can see that life expectancy nearly differs by a scale of 20. Now we need to verify if this distinction within the value of life expectancy in South Africa and Ireland is actually legitimate and not simply by pure likelihood. Our subsequent step is to match the life expectancy of two locations and perform the t-test to examine if the comparison follows a Null Hypothesis or an Alternate Hypothesis.
Check out for Data Science Course in Bangalore
The values of the parameters need to be such that the probability of the predictions that occur have to be maximum compared to the data values that were really observed. This means the distinction between the actual and predicted value needs to be much less, thereby reducing the error and growing the accuracy of the predictions. If you're wanting to be taught more about statistics and how to mine massive data sets for useful information, information science may be right for you. Competency in statistics, pc programming, and data know-how may lead you to a successful profession in a wide range of industries. Data scientists are wanted virtually all over the place, from health care and science to business and banking. Statistics, as a tutorial and skilled self-discipline, is the gathering, evaluation, and interpretation of information.
It can also import data from Microsoft Excel, Microsoft Access, MySQL, SQLite, Oracle, and different applications. Now that we’ve seen the stats and math behind Descriptive evaluation, let’s attempt to work it out in R. Descriptive Statistics uses the info to supply descriptions of the population, both through numerical calculations or graphs or tables. Although the purpose of each of these analyses is to provide results, Quantitative evaluation offers a clearer image hence making it crucial in analytics. The population is the set of sources from which information has to be collected. Several Statistical functions, rules, and algorithms are carried out to analyze raw information, build a Statistical Model and infer or predict the result. Statistics is a Mathematical Science pertaining to data assortment, evaluation, interpretation, and presentation.
To beneath the characteristics of basic inhabitants, we take a random pattern and analyze the properties of the sample. We take a look at whether or not the identified conclusion represents the population accurately and at last, we interpret their results. Whether or to not accept the speculation relies upon upon the proportion value that we get from the speculation. Descriptive Statistics helps arrange knowledge and focuses on the characteristics of data offering parameters. Statistics is used to process complex problems in the true world in order that Data Scientists and Analysts can search for meaningful trends and changes in Data. In easy phrases, Statistics can be utilized to derive meaningful insights from data by performing mathematical computations on it.
This is an alternative to frequency statistics, which is usually used to calculate chances. The probability principle is a branch of mathematics that measures the chance of a random event occurring, in accordance with Encyclopedia Britannica. A random experiment is a physical state of affairs with an outcome that can’t be predicted until it’s noticed. Probability is a quantifiable number between zero and one that measures the probability of a sure event occurring. The chance of flipping a coin is 0.5 since a touchdown on heads or tails is equally probable. On the opposite side, data science is the fad of the new and without it, many supreme selections could not have been attainable.
In the abstract of the model, notice one other necessary parameter called the t-value. A bigger t-value means that the alternate speculation is true and that the difference in life expectancy just isn't equal to zero by pure luck. Statisticians use hypothesis testing to formally examine whether the hypothesis is accepted or rejected. Hypothesis testing is an Inferential Statistical technique used to determine whether there may be sufficient proof in a knowledge pattern to deduce that a sure situation holds true for a whole population. If we want to find out the most typical type of cylinder amongst the population of automobiles, we will verify the value which is repeated the most number of times.
For extra big data, use regression and quantile regression are used. Inferential Statistics is extra prevalent in learning human nature and understanding the characteristics of the dwelling. To analyze the trends of a basic population, we take a random sample and study its properties.
Random samples are normally fairly representative since they don't favor certain members. If we put these two subsets one over the opposite, we might get a significant overlap as 9 to eleven – That’s why the median for both field plots are about 9-11, for the explanation that median negates the outliers. Finally, we come to essentially the most underused parameter, which is the mode. In a set of, the mode is 1, but it’s not close to the average, which is three. Thus, the implied is the quantity around which the whole data is unfolding out. However, mathematically, “they mean” is outlined because the sum of all the numbers in a dataset, divided the depend on numbers.
Navigate to:
360DigiTMG - Data Science, Data Scientist Course Training in Bangalore
No 23, 2nd Floor, 9th Main Rd, 22nd Cross Rd, 7th Sector, HSR Layout, Bengaluru, Karnataka 560102
1800212654321
Visit on map: Data Science Course in Bangalore
Yorumlar