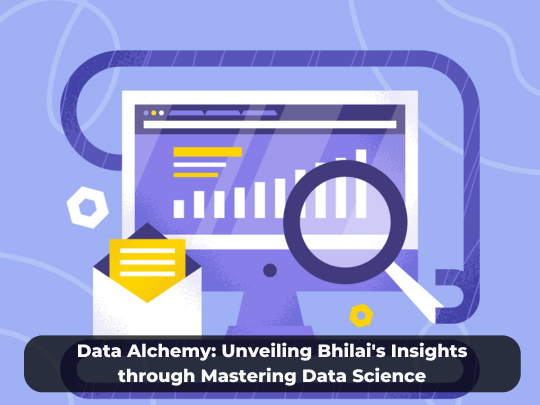
In the heart of Chhattisgarh lies Bhilai, a city steeped in industrial heritage and pulsating with vibrant energy. Amidst the clang of steel and the hum of machinery, Bhilai harbors a wealth of untapped potential, waiting to be unearthed through the lens of data science. Welcome to the realm of data alchemy, where the transformation of raw data into valuable insights holds the key to unlocking Bhilai's true essence and propelling it into a new era of prosperity and innovation.
Introduction: The Promise of Data Alchemy in Bhilai
Data alchemy is more than just a metaphor; it represents the transformative power of data science to turn raw data into actionable insights, much like alchemists of old sought to turn base metals into gold. In Bhilai, a city synonymous with steel production and industrial prowess, the application of data alchemy holds immense promise across various sectors, from manufacturing and logistics to healthcare and urban planning. By mastering the art of data science, Bhilai can uncover hidden patterns, optimize processes, and make informed decisions that drive progress and development.
The Landscape of Bhilai: A Tapestry of Industry and Innovation
Bhilai's story is one of resilience and evolution, shaped by its rich industrial heritage and forward-looking vision. Home to one of India's largest steel plants, Bhilai Steel Plant (BSP), the city has been a cornerstone of the nation's industrial growth since its inception in the 1950s. Over the years, Bhilai has diversified its industrial base to include sectors such as manufacturing, engineering, and services, reflecting its adaptability and dynamism. Today, Bhilai stands at the cusp of a new chapter in its journey, fueled by the transformative potential of data alchemy.
Unveiling Insights: The Role of Data Science in Bhilai's Growth Story
At its core, data science is about turning data into actionable insights that drive informed decision-making and innovation. In Bhilai, where data is abundant across industries and domains, the application of data science can unlock a wealth of untapped potential. From optimizing production processes and supply chain management to predicting maintenance cycles and customer behavior, the possibilities are endless. By harnessing the power of data science, Bhilai can gain a competitive edge, enhance efficiency, and chart a course towards sustainable growth and development. Visit here to learn more about the data science course in Bhilai
Leveraging Data Alchemy in Bhilai's Industries
The industrial landscape of Bhilai presents a fertile ground for the application of data alchemy. In the steel industry, for example, data science techniques such as predictive analytics and machine learning can be used to optimize production schedules, minimize downtime, and improve quality control. Similarly, in the manufacturing sector, data-driven insights can streamline operations, reduce waste, and enhance productivity. Beyond traditional industries, emerging sectors such as renewable energy and advanced manufacturing can also benefit from the application of data alchemy to drive innovation and efficiency.
Transforming Healthcare through Data Science
In addition to its impact on industries, data science has the potential to revolutionize healthcare delivery in Bhilai. By analyzing patient data, medical records, and diagnostic images, healthcare providers can identify trends, diagnose diseases early, and personalize treatment plans. Furthermore, predictive analytics can help anticipate healthcare needs, allocate resources effectively, and improve patient outcomes. In a city like Bhilai, where access to quality healthcare is essential, the application of data alchemy in healthcare can make a tangible difference in people's lives.
Urban Planning and Smart Cities: A Data-Driven Approach
As Bhilai continues to grow and evolve, the need for smart, sustainable urban planning becomes increasingly important. Data science can play a pivotal role in this regard by providing insights into population trends, traffic patterns, and infrastructure needs. By analyzing data from sources such as sensors, satellite imagery, and social media, urban planners can make informed decisions about infrastructure development, transportation systems, and public services. The result is a more livable, efficient, and resilient city that meets the needs of its residents while minimizing its environmental footprint.
Building a Data-Driven Ecosystem in Bhilai
Realizing the full potential of data alchemy in Bhilai requires more than just technical expertise; it requires the development of a robust data-driven ecosystem comprising skilled professionals, infrastructure, and supportive policies. Investing in data science education and training programs can cultivate a talent pool equipped with the skills to harness the power of data. Furthermore, establishing data repositories, cloud infrastructure, and secure data sharing mechanisms can facilitate seamless access to data while ensuring privacy and security. Additionally, fostering collaboration between industry, academia, and government can catalyze innovation and drive the adoption of data science across sectors.
Conclusion: The Dawn of a New Era
As Bhilai embarks on its journey of data alchemy, it embraces a future filled with possibilities and promise. By mastering the art of data science, Bhilai can unlock insights, drive innovation, and propel itself into a new era of prosperity and progress. From optimizing industrial processes and improving healthcare delivery to planning smart, sustainable cities, the applications of data alchemy are limitless. As Bhilai harnesses the transformative power of data, it paves the way for a brighter, more resilient future for generations to come.
Kickstart your career by enrolling in this data science certification in Bhilai
Navigate To:
360DigiTMG - Data Science, AI, Data Analytics, IoT, PMP, Digital Marketing, Cloud Computing, Cyber Security Certification Course Training Bhilai
360DigiTMG - Door No: 244, Zonal Market,Sector 10, Bhilai, Dist-Durg,
Chhattisgarh - 490006
Email: bhilai@360digitmg.com
Phone:+91 98866 28363/ +91 99816 17903
Get Direction: data science career